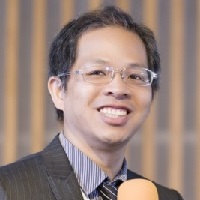
The New Era of AI Agents
Prof. Wen-Huang Cheng (University Distinguished Chair Professor; Fellow, IEEE&IET)
National Taiwan University, Taiwan
ABSTRACT
In recent years, AI development has progressed from the traditional "software" era into the transformative era of "large-scale AI models." During the software era, AI systems primarily relied on hardcoded rules, limiting their ability to make nuanced judgments or adapt to dynamic situations—traits inherent to human cognition. In contrast, today’s large models can process unstructured inputs such as natural language and produce diverse outputs, including text, images, and more. This dynamic capability brings AI closer to human-like reasoning and sets the stage for the next evolutionary step: the era of AI Agents. AI Agents are no longer static programs governed by predefined rules. Instead, they leverage large models to continuously learn, self-improve, and flexibly adapt to evolving environments and contexts. This talk will offer an in-depth overview of the foundational technologies and application scenarios driving AI Agent development. It will also explore current trends and key challenges, providing actionable insights and inspiration for technology innovators.
SPEAKER BIOGRAPHY
Wen-Huang Cheng is a University Distinguished Chair Professor in the Department of Computer Science and Information Engineering at National Taiwan University and a Visiting Professor at the Korea Advanced Institute of Science and Technology (KAIST). His current research interests include multimedia, computer vision, and machine learning. He has actively participated in international events and played significant leadership roles in prestigious journals, conferences, and professional organizations. These roles include serving as Editor-in-Chief for IEEE CTSoc News on Consumer Technology, Senior Editor for IEEE Consumer Electronics Magazine (CEM), Associate Editor for IEEE Transactions on Pattern Analysis and Machine Intelligence (TPAMI) and IEEE Transactions on Multimedia (TMM), General Chair for ACM MMAsia (2023), IEEE ICME (2022), and ACM ICMR (2021), Technical Program Chair for ACM MM (2025), ACM ICMR (2022), IEEE ICME (2020), IEEE VCIP (2018), Chair for IEEE CASS Multimedia Systems and Applications (MSA) technical committee, and governing board member for IAPR. He has received numerous research and service awards, including the NVIDIA Academic Grant Program Award (2025), the 2024 Best Paper Award of IEEE Consumer Electronics Magazine, the Best Paper Award at the 2021 IEEE ICME and the Outstanding Associate Editor Award of IEEE TMM (2021 and 2020, twice). He is an IEEE Fellow, IET Fellow, and ACM Distinguished Member.
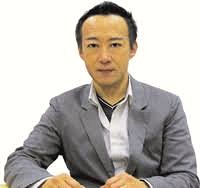
Designing Optimal Latent Space toward Knowledge Extrapolation
Prof. Kenji Yamanishi
Tokyo University, Japan
ABSTRACT
Recent success of AI/machine learning technologies is largely due to the embedding of the original data into a latent space, where we can extract essential features necessary for data mining tasks such as prediction, classification, and clustering. There are critical issues 1) how should we design an optimal latent space, depending on the nature of the data? and 2) how should we utilize the latent space not only for learning in a classical sense, but also for knowledge extrapolation? This talk introduces recent advanced technologies for addressing these issues. As for 1), I show a novel methodology for optimally selecting the kind of space (Euclidean or non-Euclidean), dimensionality, and curvature for the latent space. I show that they are obtained within a unifying framework of the minimum description length (MDL) principle. As for 2), I show that a novel but reliable knowledge can be generated by modeling the embedded data with Gaussian mixture model and then manipulating it adequately on the basis of the MDL principle. Both 1) and 2) are widely applicable to the areas including graph mining and generative AI.
SPEAKER BIOGRAPHY
Kenji Yamanishi is a professor in the Graduate School of Information and Technology at the University of Tokyo. He received the degree of doctor engineering from the University of Tokyo, 1992. He used to work for NEC Corporation from 1987 to 2008, and his final position was a fellow. He joined the University of Tokyo in 2009, and was an associate dean of the graduate school(2019-2021). His current research interests include information-theoretic machine learning, data mining, and computational ophthalmology. Specifically, he is a pioneer of learning theory based on the minimum description length (MDL) principle. He has also contributed to the area of anomaly/change detection and text mining with their applications to industries. He has been working as an area chair or a regular or senior program committee member of ACM SIGKDD(Knowledge Discovery and Data Mining) for years, an associate editor of KAIS(Knowledge and Information Systems) and IJDSA(International Journal of Data Science and Analytics), an editorial board member of Entropy, and a honorary chair of WITMSE (Workshop on Information Theoretic Methods for Science and Engineering). He is a fellow of IEICE(Institute of Electronics, Information and Communication Engieers) and a senior member of IEEE. He obtained several awards including IBM Faculty Awards, Fuji-Sankei Businetss Award, etc. He is an author of the book: “Learning with the Minimum Description Length Principle” published by Springer in 2023.
Nurturing Successful Intellectual Styles for Effective Education Technology and Learner Growth
Prof. Li-fang Zhang
The University of Hong Kong, Hong Kong
ABSTRACT
Intellectual styles refer to individuals' preferred methods of utilizing their abilities. In an era increasingly shaped by information technology (IT), the importance of fostering creativity and embracing diversity has grown significantly. This talk begins with a brief introduction to the field of intellectual styles, discussing their nature and significance. It then presents primary research evidence highlighting the crucial role of a diverse range of intellectual styles – particularly creativity-generating styles, referred to as “successful intellectual styles” – in online learning environments, while also considering relevant findings from offline contexts. The talk concludes by proposing practical implications of these research findings for students, educators, and IT designers, aimed at enhancing the effectiveness of educational technology and fostering student learning and development.
SPEAKER BIOGRAPHY
Li-fang Zhang is a Professor of Psychology and Education at The University of Hong Kong. She has (co)authored over 150 peer-reviewed journal and encyclopedic articles and published dozens of academic book chapters and books, including two monographs with Cambridge University Press. She is the editor-in-chief of the Oxford Encyclopedia of Educational Psychology and associate editor of Educational Psychology. Moreover, she serves as a consulting editor for the Journal of Educational Psychology and on several editorial boards in psychology and education.